Talk is highly detailed. Everything we say can convey meaning, and we don’t often chat in silent environments. This means that for machines to parse human speech, they need to collect as much information as possible, and separate the desired signal from the background noise.
While ASR has improved, there’s still plenty of room for improvement – for example, conversational assistants still struggle with interruptions, which are a normal component of conversations.
One company that’s done incredible things with natural language understanding is Action AI. CEO, John Taylor, spoke all about it on VUX World’s stage at The European Chatbot & Conversational AI Summit in Edinburgh in 2023.
Customers still want to call you
It’s still common for businesses to use call centres, and rather than being replaced by chatbots and voice assistants, phone lines are also being automated so that they serve user’s needs better.
“I’m sure voice is not going away from a customer service perspective. It’s still about 70% of all contacts. Here are a few of the call centre challenges: calls are expensive to handle, customer service isn’t always great via voice, long wait times, difficulties getting first call resolution when you get through, and you don’t always get through to the right person every time.” John Taylor, CEO, Action AI
There are also specific challenges when automating voice detection, transcription and understanding; machines often struggle with detecting the start and end of speech, handling interruptions, dealing with disfluencies (e.g. stutters or repetitions), and recognizing background noise.
Every domain has bespoke needs
Action AI has aimed to solve these problems by tailoring their solution to each client’s specific domain, such as banking or utilities, as they each have different needs.
But while each domain may use specific language and have certain needs, there’s commonalities among many customer service calls.
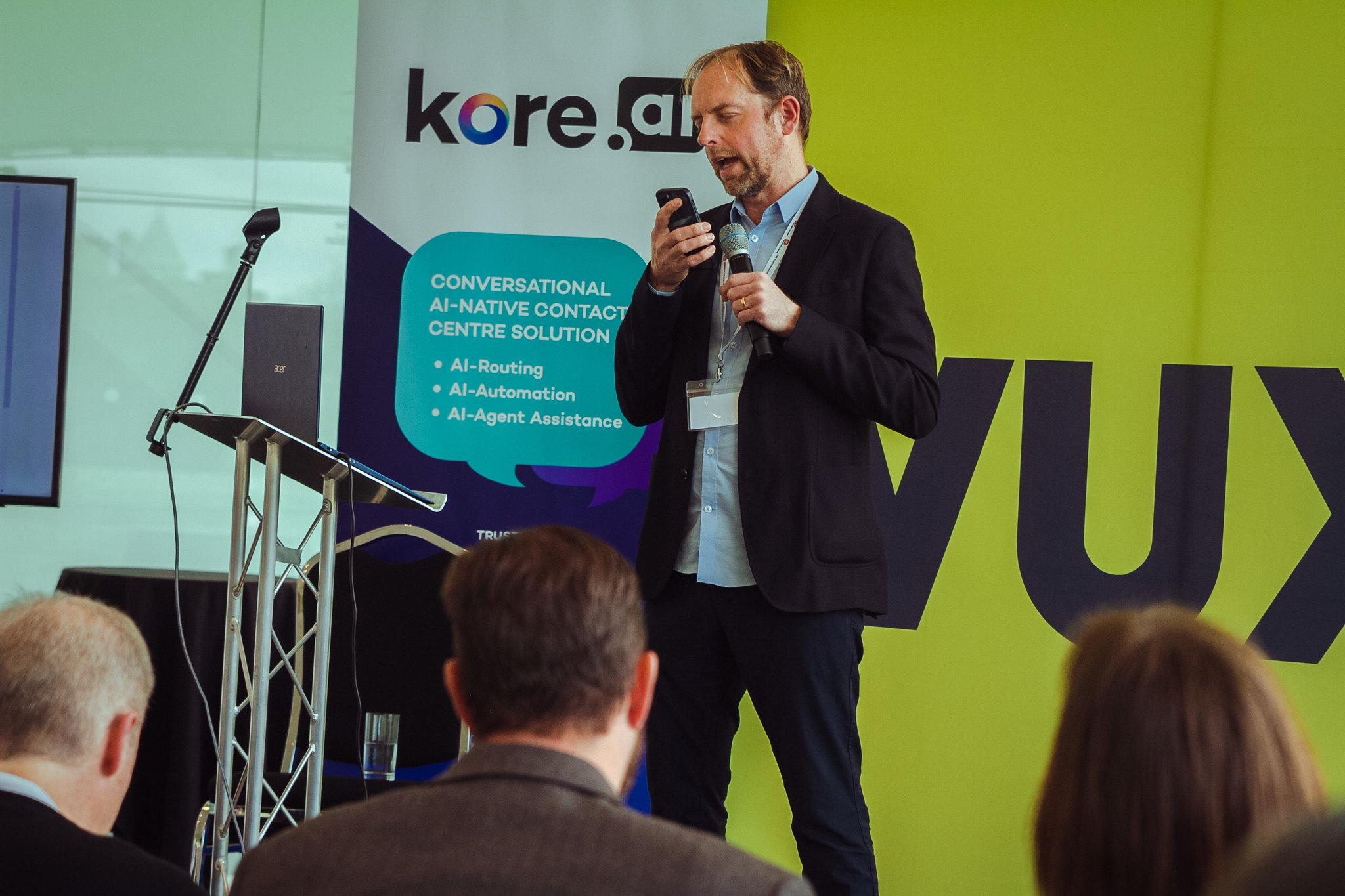
John Taylor on the stage of VUX @ The European Chatbot and Conversational Summit 2023 in Edinburgh
End of speech detection challenges
One of these is deciding when the customer has finished speaking. Consider this example; a customer was asked for their ID number and they said “oh, my ID number – Let me find that for you,” and then they stopped talking while they went to look for it.
Often, silence is used as a marker to detect the end of an input. In other words, the customer’s unfinished sentence might be taken as their entire utterance, and the assistant would attempt to act on their words, which would lead to errors as they’ve not yet given the information they were asked for.
The challenge is in creating systems that are aware that the customer hasn’t stopped talking yet! Soon after the silence they’ll start talking again and say something like, “got it – my ID number is 123…”
Action AI have considered such scenarios, and their technology will wait for the customer to return and give the vital information.
To enhance the system further, they incorporated GPT models, which allows them to parse complex user utterances. Traditional NLU systems are designed to respond to pre-decided user inputs, whereas LLMs can parse unexpected inputs to glean the user’s intent.
A voice UI should remove friction
Simply, people just want to talk to machines like they would talk to another person. They expect it to work.
For machines, this means they need to be able to glean meaning from diverse and complex utterances, as expressed by diverse and nuanced people!
When John described his speech he said, “I’ll be verbose. I’ll go off subject, I’ll change my mind. I’ll stutter. I’ll be human. That’s okay. It’s valid for me to be human.”
That applies to every single person, and every single customer. Our goal is for machines to be able to understand everyone better.
According to Action AI, that needs domain-specific training and advanced tools to ensure a seamless and effective customer experience.
Watch John’s entire presentation for live demos of Action AI.