While preparing this blog I had an uncanny moment.
I was reading transcripts of Kane’s interview with Rasa’s Alan Nichol and simultaneously wondering how accurate some of the transcription was. Was the ASR used for the transcription accurate? It’s important because you have to be
confident about what people are saying to get what they really mean.
It goes deeper too. I read the transcript first rather than listening to the entire interview because it allows me to jump right through the interview and find the juicy bits. Had I been listening to the hour-long interview, pausing, replaying and reviewing the audio it would have taken me many hours just to get the jist of the conversation. I only use the audio to confirm what I’ve read. I may be sure of the audio accuracy (it’s the source of truth) but it would have taken much longer.
The thoughts I had matched perfectly with some of the things Alan and Kane were discussing; how confident we are about NLU, how we scrutinise data, and when we should trust our own brains?
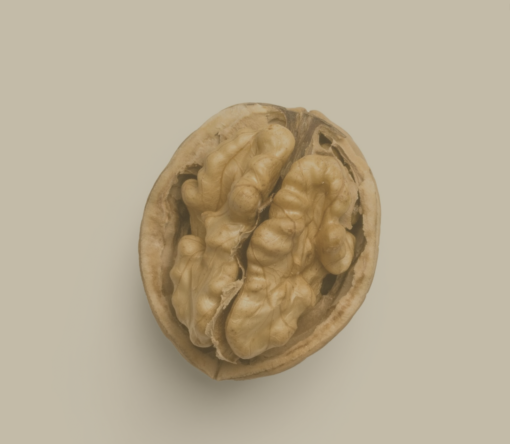
Will AI see a walnut or a brain?
Taking your best guess at what users say
All conversational designs are hypothetical. You’re guessing what people will want, you’re guessing how they’ll ask for it, and you’re guessing how well you’ll be able to understand what they will say.
That’s a precarious position to be in. If things go wrong it could be costly.
To mitigate that risk there are strategies available.
Start with data
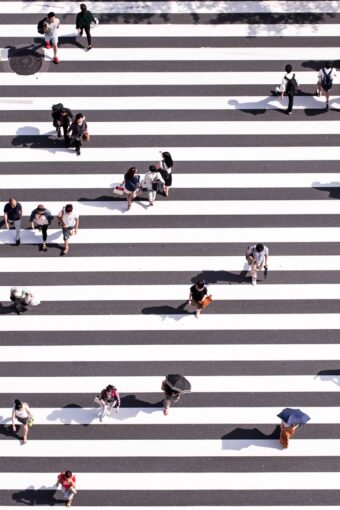
look long enough and you’ll find patterns
Alan recommends returning to your transcripts, as they are the source of truth – they tell you what users want and how they say it. Your ability to process the information contained within is unparalleled because you understand cTrain your model with a data set that represents the problem you’re trying to solve. Get real data from real customers and work with that.
As Alan says, “the problem you need to solve is – how can you create a dataset that best represents how your users are actually talking?”
But it’s not just about letting the machines crunch the data and spit their results out at you. You should also familiarise yourself with that data. You won’t be able to read everything as quickly as the machine does, but you will be able to draw conclusions that a machine cannot.
For example, if a user says “I took the dog for a walk and bought new shoes” in a single sentence, it could be chunked into a single unit of data, as if ‘taking the dog for a walk’ and ‘buying new shoes’ are naturally connected. But they might just be talking about their day, and didn’t mean those two events were connected.
We humans will work that out fairly quickly, but to a machine it’s not so simple. Our brains are incredible at finding patterns, too.ontext.
The intensity of intents
See the image? That’s the transcript of Alan talking, and the reason I started to wonder about transcription accuracy. Transcripts speed up my work incredibly, but sometimes human language just isn’t so easy to capture. He wasn’t saying “intense” – he was saying “intents”.
Despite the massive challenge of transcribing what people say, it’s only one part of the problem. What did Alan mean? That’s
where conversational AI gets gritty. That’s right at the centre of everything we do.
Dealing with ambiguity
An airline bot may ask a user “was the flight delayed?” to which the user replies “unfortunately.”
In that context ‘unfortunately’ means ‘yes’. So you could mistakenly add ‘unfortunately’ as a sample utterance to the intent; ‘yes’.
Then in the same experience, the bot could ask “would you like to book more flights?” to which the user replies “unfortunately, no.”
Does the addition of the word ‘no’ always mean negative, while ‘unfortunately’ said by itself always means positive? If you went ahead with that hypothesis you could be on very unstable ground as both yes and no intents could be triggered by the same word, when the only differentiator is a tiny and easily misheard ‘no’.
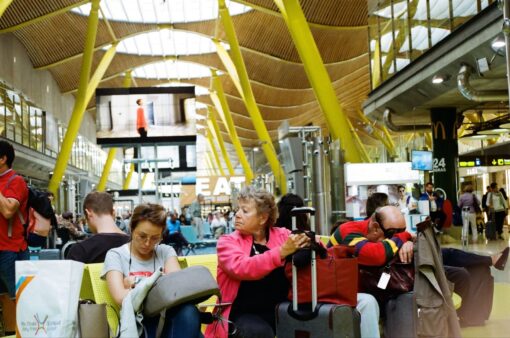
You might not hear “no” and that could make or break the experience
What we say and what we mean – it’s all about context
Knowing what users mean and what they want gives you superpowers. That is the most useful piece of data because, if you know their intention, you can provide the steps to take them to their goal.
Intents are discussed often within the industry, because many people rightly wonder if there is a better way to structure conversations with machines. Perhaps there is a better way, but right now intents are ubiquitous. Intents allow you to zero in on the things your user might want to do with your experience. Add too many intents and it becomes unstable, add too few and it might not be very helpful. Sadly, there’s no rule about the perfect number because it depends on what you’re trying to build.
But if you build your experience around real customer data, keep paying attention to how accurately you’re understanding your users, and keep checking how you map their words to what they want, then you’re on your way. Keep paying attention to the users and their problems, and they’ll tell you what they need.
As NASA’s Gene Kranz said, “work the problem”.
This article was written by Benjamin McCulloch. Ben is a freelance conversation designer and an expert in audio production. He has a decade of experience crafting natural sounding dialogue: recording, editing and directing voice talent in the studio. Some of his work includes dialogue editing for Philips’ ‘Breathless Choir’ series of commercials, a Cannes Pharma Grand-Prix winner; leading teams in localizing voices for Fortune 100 clients like Microsoft, as well as sound design and music composition for video games and film.