We’ve put together the DITAT maturity model for conversational AI to show the various stages of maturity conversational applications go through on the journey to AI transformation. The model shows the types of enterprise use cases conversational AI can solve for, as well as the value each can bring to customers and your business.
As you’re thinking about your customer experience automation strategy, business leaders need to consider how they should prioritise and scale automation initiatives. You can use this model to sense-check your strategy, find a place to start, understand how to develop maturity, as well as to help you make stronger decisions about your CX automation approach.
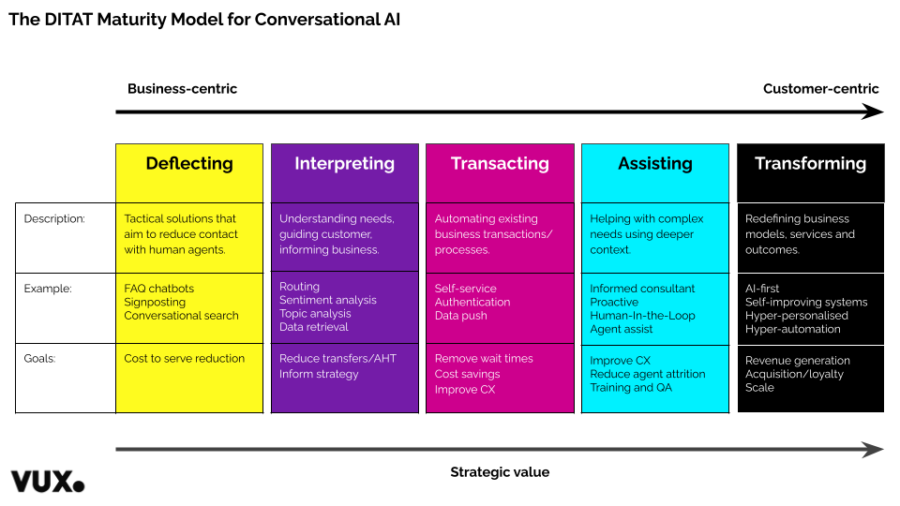
The DITAT model, so called because, if you use it, you’ll quickly DITATch yourself from the status quo and head straight into transformative territory.
How it works
Starting from left-to-right, the model shows business-centric solutions to the left. These are solutions that are predominantly implemented by focusing on business problems, rather than user needs. If all of your automation initiatives fall on the left-hand-side of the model, you’re taking a business-centric view of the world.
This can lead to some short term benefits, such as proving the value of AI technologies, but doesn’t add a tremendous amount of strategic value. It can also sometimes lead to solutions that customers view as ‘getting in the way’ if not implemented correctly.
As you progress from left-to-right, you start moving towards customer-centric solutions. These solutions are aimed at solving customer pain points and providing excellent customer experience.
The more of your initiatives and use cases that fall on the right-hand-side of the model, the more you’re taking a customer-first view of the world. Taking a customer-centric approach results in a higher chance of you developing solutions that meet the needs of your customers, provide better CX and ultimately lead to higher strategic value.
So let’s break down each stage of the model.
Deflecting
Most conversational automation initiatives begin here. It’s the low hanging fruit. The simple, repetitive queries that are easily automated by a chatbot or equivalent.
The aim of Deflecting use cases is to reduce contact with human agents by deflecting the customer contact away to another channel. Typically, that ‘other channel’ is a chatbot that’s geared to answering customer questions.
The aim here is that the chatbot can answer some simple questions and prevent the customer from having to talk to someone.
Deflecting use cases use content, usually hard-coded into the bot, or taken from webpages or knowledge bases, to answer customer questions. They may even simply signpost users to a web page by serving a link instead of answering a question directly. Or, they may appear in website search bars to enable users to find content using natural language queries.
The business goals for pursuing a Deflecting strategy are typically rooted in trying to reduce cost to serve. If you can ‘contain’ customers in your chatbot or IVR, and prevent them from reaching an agent, you don’t have to pay someone to handle that contact.
You can see by the language used above that this strategy is a business-centric approach. If done well, it can be valuable to customers, but it’ll only serve part of their journey.
That said, when implementing conversational AI, it’s recommended that you crawl before you walk. These Deflecting use cases will enable you to do just that. You can learn the technology, design principles, implementation and management tools and processes in a low risk environment.
Once you’ve graduated from Deflecting, you’ll move to Interpreting.
Interpreting
The aim of an Interpreting strategy is to use conversational technologies to gather insights on customer needs and business problems in order to make better strategic decisions, or to be able to answer more complex customer queries with data retrieval.
Examples of Interpreting use cases include:
- Data retrieval: identifying the intent of the user and, instead of answering a query with content, when you’re Interpreting, you’re able to retrieve information from business systems to provide more personalised answers to more complex queries.
- Routing: using conversational AI to determine what a customer wants to accomplish, and then routing them to the right agent. This can help improve first call resolution and remove the need for switchboards.
- Sentiment: analysing customer sentiment to understand whether customers are happy or angry. You can then use this information to inform agent training and quality assurance monitoring. If you’re getting fancy, you’d combine sentiment analysis with conversational automation and tailor your customer interaction based on customer sentiment. For example, if someone’s angry, perhaps you’re better off escalating them from the bot to a live agent.
- Topic analysis: using conversational intelligence to analyse conversations across channels to understand customer needs and to find issues that were previously undiscovered. For example, let’s say you receive a bunch of phone calls from customers with a specific product complaint. Rather than it staying in the heads of agents, it can be aggregated, understood, passed to business units and used to improve the product. You can also use topic analysis to put together a prioritised roadmap for automation activity.
- Matchmaking: using emotion intelligence to profile the speaking style of the customer and match them with a live agent that has the same speaking style. This has been proven to lead to better conversations.
- Feedback: there’s no better source of truth than the horse’s mouth. NLP can be used to gather and analyse feedback from customers to improve service delivery.
- Authentication: using biometrics to authenticate a customer in order to serve them quicker when they speak to an agent. This can help improve fraud detection, customer trust and reduce average handle time.
The goals for using an Interpreting strategy are predominantly to use data to inform your wider business strategy. However, use cases with Data Retrieval and Routing can have an impact on things like average handle time, call transfer rates, first time resolution and NPS.
Transacting
When you’ve mastered Interpreting, it’s time to start making some proper business and customer impact. It’s time to start Transacting.
Transacting is where you’re enabling customers to get things done via your conversational assistant. It’s where you allow customers to complete tasks. It’s the stage when a bot becomes an assistant.
This requires both the retrieval of data from systems and also the pushing of data into systems to instigate processes and workflows.
Rescheduling a delivery, booking an appointment, changing your registered address, making a purchase, cancelling or upgrading a service, taking out insurance; these are all Transacting use cases.
Most of the time, when you first start building transactional use cases, you’re building on top of the existing business processes. You’re also building on top of existing business systems and using existing APIs to facilitate automation.
Sometimes, the requirement to develop Transacting use cases can lead to the requirement for the business to create connectivity into line of business systems. However, this is still typically to enable an existing business process to be automated.
Many Transacting use cases also require authentication i.e. you need to know who the user is in order to help them. Therefore, many AI teams begin their Transacting journey by having their assistant available to people who are already logged into their mobile app or website. However, more sophisticated Transacting use cases will include pieces from your Interpreting stack, such as voice biometrics, network authentication or 2FA to identify and verify users.
The aims of pursuing a Transacting strategy are typically to a) improve customer experience and b) improve operational business performance. When a customer has a task completed using a conversational interface, these can be some of the most surprising and delightful experiences that exist.
Automating transactions also can lead to potential cost savings for the business, as well as an increase in service availability, scale, reduction in wait times and better customer experience with live agents (because live agents can now serve customers with more complex needs).
Assisting
When you’ve proven the value of Transacting, you’ll develop your maturity further and begin Assisting.
An Assisting strategy is where you begin to help users with deeper, more complex needs. Needs that the user might not even realise they have themselves. This builds on level 4 and 5 of Rasa’s 5 levels of conversational AI. It requires your assistant to take a consultative approach, helping the customer arrive at a solution, and means you’ll need a more sophisticated approach to conversation design and natural language understanding.
When you’re Assisting, you’re also using context and information you already have about your user to make the experience more proactive and personal. For example, recognising that your caller has recently placed an order, and preempting the conversation by prompting “Are you calling about the order you placed yesterday?”
In order for your assistant to develop these advanced capabilities, you not only need further connectivity into line of business systems, but also the ability to have humans working alongside your assistant to step in when required. For example, if your assistant doesn’t recognise an intent, handing over to a human agent who’ll classify the intent in real time, before handing the conversation back to the assistant to continue the conversation.
Another component to Assisting is using technologies such as Agent Assist. This is where a companion assistant listens in to calls between customers and agents, recognising the intent of the caller and prompting agents with next-best-actions, conversation starters, prompts, coaching or even facilitating transactions.
By bringing Transacting use cases to the fingertips of agents, joining together many business systems and processes, and making it available to agents via a single interface during the call in real time means that agents can focus on the conversation and the assistant can do the processing of transactions. Here, you’re Assisting customers, and internal staff.
The aim of an Assisting strategy is to provide next-level customer experience and to improve NPS and CES (Customer Effort Score) and to streamline agent workloads, reduce agent attrition, and improve training, quality assurance and consistency.
Transforming
When you enter the Transforming phase, you’re really letting the conversations that you’re having with customers guide and shape the business processes and systems that underpin them.
You’re now able to accomplish things that humans alone can’t do. You’re operating at the bleeding edge of customer-centricity, and everything you do is geared at making people’s lives easier.
Here, you’ll be making your AI assistant available in all conversational channels, handling a wide breadth of end-to-end processes. Processes that have been redesigned specifically around the needs of the conversation. This is what we at VUX World call Conversational Service Design.
You’ll have self-learning and self-improving systems that can adjust based on the AI assistant’s performance or user behaviour. For example, let’s say you get a 10% increase in responses to text messages than you do emails; then you’d want your assistant to begin to prefer texting people, rather than emailing. This is something that the system should be able to do itself, without human involvement.
Transforming use cases are hyper-personalised to the point where it’s possible that no two conversations are the same. If you’re able to authenticate a user based on the number they’re calling from, dip into your CRM to find their last interaction, recognise that they’ve called twice today already, show empathy and apologise for the hassle, issue the refund they asked for, then cross-sell an upgraded product, you’re now not only hyper-personalising the experience, you’re generating revenue and transforming your business.
This requires you to rethink your business processes, redesigning them from the ground-up around the customer journey. Then, your technology behind-the-scenes needs to be architected to deliver on that customer journey. Finally, you need the skills, and the organisational culture to bring all of this to life. Welcome to Conversational Service Design.
The goals of pursuing a Transforming strategy are to create long term strategic differentiation, to prevent strategic drift, to create new value streams, new products and services, new operating models and next-generation customer experience.
A Transforming strategy will enable you to scale without recruiting heavily, serving more customers with less resources, build a culture of innovation and constant improvement, and to future proof your business.
Near-term aims include revenue generation, customer acquisition, retention, customer experience improvements and cost savings and much more.
Time to build your strategy
You can plot your current use cases and roadmap across this model to understand your automation and CX improvement maturity, and to generate ideas and inspiration for how to level-up your plans.
For more information on how to practically apply the model, and to learn more about how Conversational Service Design can transform your customer experience and business results, feel free to reach out and book a free, no obligation call with me.